Gender Bias in AI. Interview with Philosopher of Technology Galit Wellner
With AI systems being used in various domains, such as medical diagnosis, cybersecurity, and facial recognition systems, we should not forget that datasets and algorithms are not just neutral amplifications of our decision-making processes.
Kevin Rändi, a junior researcher and doctoral student at Tallinn University, interviewed Dr. Galit Wellner, a philosopher of technology and an academic advisor for the AI Regulation Forum of the Israeli Ministry of Innovation, Science and Technology. Wellner highlights how gender biases—prejudices to prefer or misrepresent one gender—in AI exist and pose a problem for multiple professional and everyday contexts where we expect AI to provide a truthful sense of the world.
As AI-based decision-making has gained momentum, there are now numerous examples of AI exhibiting bias, such as a machine preferring male names or mirroring racism. Galit, can we say where these biases are rooted in AI, or what exactly is to be blamed for such decision-making?
Important works such as Cathy O’Neil’s Weapons of Math Destruction, Caroline Criado-Perez’s Invisible Women, and Catherine D’Ignazio & Lauren F. Klein’s Data Feminism have all highlighted how algorithms and data do harm and injustice toward women. Some data scientists “blame” the data for being biased, explaining that it simply reflects the world. Cathy O’Neil (Weapons of Math Destruction), and Catherine D’Ignazio & Lauren Klein (Data Feminism) have shown how the mere selection of a certain data source already reflects potential biases. Think of a popular open dataset such as the Enron emails. Without context (bankrupt company, managed by mostly white males), a data scientist might be surprised that the system trained with this presumably neutral dataset is heavily biased. Sometimes the bias arises in the training process in which greater weight is given to some parameters and less to others, thereby reflecting implicit biases. In severe cases, the biases are explicit, but so far it seems that explicit bias is the exception rather than the rule.
What are the research disciplines at the intersection of feminism and technology? How do you position yourself regarding these issues in your work?
The works of O’Neal and D’Ignazio & Klein inspired my job a lot, and many of the examples I use in my papers were brought by these authors. What I like about their works is that they practice feminism in a broad sense, so that it is relevant to everyone concerned about inequality, women and men alike. Interestingly, these books were published before the launch of ChatGPT, and managed to remain relevant— even becoming more so!—after November 2022, when OpenAI opened ChatGPT to the public with no limitations.
Regarding the question of research disciplines, the first is, of course, gender studies. However, with AI we need a good understanding of technologies that will avoid the utopian and dystopian approaches frequently found in the humanities. We also need to realize that technologies are never neutral, and they do something, related to many aspects of human values and worldviews.1 So, the disciplines I’m using are philosophy, sociology, anthropology, and psychology. I usually refer to these disciplines indirectly through interdisciplinary frameworks that bind them together – mostly Science, Technology, Society Studies (STS2) and postphenomenology3 which is a branch of the philosophy of technology originally developed by Don Ihde.
Broadly speaking, phenomenology is the study and description of how the meaning of something is formed in our consciousness, with a focus on the embodied and contextual characteristics of an experience. As a school of thought, it remains relevant beyond philosophy. However, how would you define the postphenomenological branch of the philosophy of technology? What does it reveal about the given issue?
My concerns regarding biased algorithms are mapped along a theoretical framework known as postphenomenology. As its name implies, it has deep foundations in phenomenology (as well as pragmatism)4. It allows me to study the experiences of people who use certain technology. In AI, people’s experiences are often narrowly considered in the technical sense of “UI” (User Interface), thereby focusing only on the immediate, short-term interactions between humans and computers.5 Postphenomenology enables me to think of the experience before and after the mere interaction with the technology.
We also need to realize that technologies are never neutral, and they do something, related to many aspects of human values and worldviews.
It allows me to systematically analyze digital technologies and their impacts on users and society. First are the aspects related to the body and senses – how we move in the world, and what we can and cannot sense. For example, if we want to design an app that counts steps to ensure we move enough daily, we should consider that the length of a step varies from body to body and is usually shorter for women. As per the senses, using AI as a search engine is, for me, equivalent to a way of “seeing” and “hearing” the environment, so I would expect to see a true picture of the world. Data does not always provide it, as it usually results from long historical social processes that are likely biased. In a perfect world, a video app will recommend clips made by people of all genders and colors to the user, but I’m not sure this is what you are likely to get today.
The next aspects are related to meaning generation and interpretation of the world, or referring to the technology as if it were another person, or treating it as part of the background, when technologies remain out of direct sight or use. In my work, I have detailed these four aspects, body and senses, meaning generation and interpretation. I also tried to develop additional relations. I believe these can help policymakers to ensure their regulation covers as many aspects as possible. A consistent analysis of these and other human-technology relations can improve the visibility of future technological developments. Consequently, the slow regulatory processes can be better aligned with the very fast technological changes.
Indeed, in 2022 you proposed two categories for policy recommendations to mitigate gender bias in AI: transparency and human-in-the-loop. Could you elaborate on these ideas?
Transparency can be practiced today on various levels: from revealing the sources for the training datasets to the logic of the AI system and the considerations implemented during the training (what is considered right or wrong), to sharing the cases where the system provided biased results (a “me too” logic). By human-in-the-loop, I proposed an “ombudsman” mechanism that ensures the biases detected by the users are dealt with by the companies who develop and run the algorithms.
I examined five solutions but detailed only the two mentioned in the policy recommendation part. The other three were:
-
- Avoiding any reference to gender in the dataset. This proved to be inefficient as proxies can infer the gender in many cases.
- Algorithms designed to be “anti-bias”. Currently, they are being studied in computer science as the alignment problem.
- Developing machine education. This one is the least developed and may have the biggest potential. It means that machine learning should be complemented with educational logic intended to produce a “moral compass”.
“Machine learning” denotes a process based on a certain mode of teaching where a human developer or data scientist serves as a “teacher” who trains the algorithm to recognize which parameters are relevant. The idea of “machine education” builds on a different logic based on self-reflection and forecasting several possible scenarios for various societal settings. Our challenge is to develop this technique for algorithmic education while also educating the users, i.e. people, in AI skills and literacy.
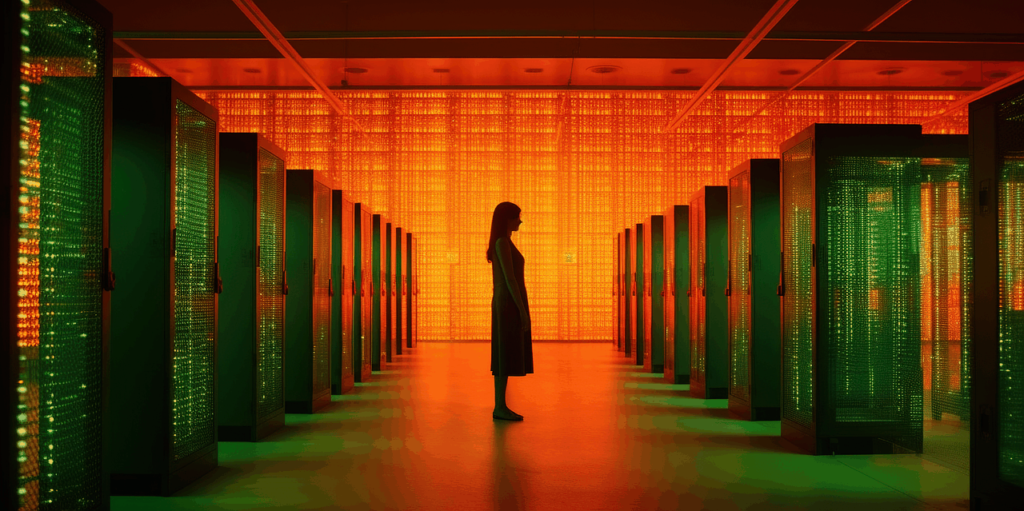
Image by Jorge Franganillo from Pixabay.
These various ways in which technologies relate to our biases and further shape them, together with your policy recommendations, indicate the specific problem of opacity. This problem seems even more significant as AI decisions can target groups of people on a larger scale. If we nevertheless must live with some degree of opacity in algorithms due to technical limitations, could we still develop more inclusive ways of relating to AI? In which ways could social, cultural, or political aspects be relevant to how we develop better relations with AI?
This is a very important question and a true challenge not only to technology developers but to all of us as a society. The technical limitations can be resolved, but the commercial considerations are subject to different mechanisms. This is where the philosophy of technology in general, and postphenomenology in particular, is trying to steer policy making. Today, even the mere probability of experiencing a bias is hidden behind the walls of intellectual property law and obscure contracts. The EU has been trying to demolish these walls first with the GDPR and more recently with the AI Act. The companies who develop AI systems should now expose the risks they have identified, and in my view, this is an important step forward in the fight for transparency.
You mentioned the AI Act, a recent European regulatory framework for artificial intelligence. As I know, you have recently published a paper on the topic. What should be known about the AI Act regarding gender equality? Does the regulation effectively address gender bias in the long run?
The gender bias was very visible when WhatsApp instructed me to change my Hebrew sentences from female to male form – even when the sentence was grammatically correct!
Gender equality is a foundational principle of the AI Act though not always directly referred to as such.6 It is good to see a direct reference to non-discrimination in the recent AI Convention by the Council of Europe, released on 17 May 2024. Article 10 urges the parties to “adopt or maintain measures to ensure that activities within the lifecycle of artificial intelligence systems respect equality, including gender equality, and the prohibition of discrimination…” The current regulation in the EU is open-ended to ensure it is relevant for various forms and modes of gender bias in AI. Only time will tell if it is “future-proof” enough.
You have also written on cell phones from the perspective of the philosophy of technology. AI algorithms have already become an integral part of the software in our smartphones. For example, AI offers tools to easily edit images and correct how the image should look when taken under different conditions. A form of this synthetic media is called a deepfake. One of the many reasons why deepfakes have been considered harmful is because technology has been used to target and sexually abuse women. What are your thoughts on these matters?
My research transition from cellphones to gender bias in AI took a slightly different path. It started with translation and autocomplete algorithms that are very common and useful when it comes to small devices like cell phones. There the gender bias was very visible to me, when, for example, WhatsApp instructed me to change my Hebrew sentences from female to male form – even when the sentence was grammatically correct!
The problem of deepfakes in the context of sexual harassment should be treated by the criminal law authorities. The police should exercise its powers and stop it, as early as in schools. In many countries the legal tools are there, they just need to be exercised in a new domain – the digital space. But we cannot stop here. The problem of deepfakes is also a threat to democratic elections, as evidenced by recent attempts by non-democratic states to muddle election processes in 2024. This should be handled by homeland security authorities, rather than the police.
During your visit to Tallinn this year, you gave an excellent presentation about the book you co-edited with Geoffrey Dierckxsens and Marco Arienti, which brings together multiple authors to explore the philosophy of imagination, technology, art, and ethics. Since we have been discussing synthetic media, I would like to delve into the possible connection between gender bias and AI-generated images and videos. Recently, AI tools have created creative visual content. How can we interpret gender bias in the context of AI-generated imagery?
In some countries, ultra-religious groups attempt to ban the display of images presenting women in public spaces.
We have seen gender bias in relatively early systems of the current AI hype, for example in AI-generated images of professionals. There have been so many examples of AI-generated images for CEOs, doctors, etc. presenting male figures only, whereas nurses would be represented mostly by female figures. This kind of bias is threatening to take us back decades. In a broader context, visual content raises visibility concerns. In some countries, ultra-religious groups attempt to ban the display of images presenting women in public spaces. It happened in Israel. It’s a shame. Publications in English are not very recent.7 It doesn’t mean the problem has gone away.8 The fear is that they use AI to augment and multiply these attempts.
It’s important to remember that gender bias can also be found in non-visual contexts, such as the job market, especially when algorithms manage employees. Studies have shown how in algorithmic markets like eBay, female traders received lower prices for the same goods, and female drivers earned less than their male colleagues. Today it is difficult to obtain such data, although I hope that with the new wave of European regulations, we will have enough transparency to detect such biases. I would like to believe that this is also of interest to the companies that operate such platforms.
Thank you so much, Galit! One last question. Estonia hopes to raise both awareness and efficiency regarding AI solutions. From your perspective, what should be kept in mind?
I wonder if efficiency is needed and if awareness suffices. In my view, the integration of AI solutions into existing governmental and business systems should result from a careful planning process that involves the public discussing risks and benefits.
- This is one of the central ideas among many approaches on technology and engineering. Technologies as designed artefacts “do something” as in they are not just instruments, but can contain all-too-human views on politics (e.g., Langdon Winner, “Do Artifacts Have Politics?”, Daedalus 109, no. 1 (1980): 121–36.)), shape our morality (Peter-Paul Verbeek, Moralizing Technology: Understanding and Designing the Morality of Things (Chicago ; London: The University of Chicago Press, 2011)), and play a part in our value-based transformations (e.g., Galit Wellner, “Digital Cultural Sustainability”, in Sustainability in the Anthropocene: Philosophical Essays on Renewable Technologies, ed. Róisín Lally, Postphenomenology and the Philosophy of Technology (Lanham, Maryland: Lexington Books, 2019), 135–49.)
- Also known as Science and Technology Studies.
- Postphenomenology is a branch of philosophy of technology that investigates the numerous ways humans use technologies and make sense of the world with technologies. It proceeds from the recognition of humans as embodied and cultural beings whose everyday experiences are shaped by technologies, everything ranging from eyeglasses to digital voice assistants. See also another recent interview conducted by Estonian philosopher Ave Mets from Tartu University discussing the importance of postphenomenology and care ethics with Robert Rosenberger: Leida | Technologies are Non-Neutral: Postphenomenology and Issues of Care
- Pragmatism puts emphasis on the material aspects, habits, and situational context that determine a particular technology use. The originator of postphenomenology, Don Ihde, criticized phenomenology as being too subjectivist and inclined towards the “consciousness language”. See: Don Ihde, Husserl’s Missing Technologies (New York: Fordham University Press, 2016), 96.
- The user interface is an instance of what the user sees that allows them to effectively interact with the computer. It includes all the graphical and non-graphical ways of interacting, such as clicking the buttons, giving a voice command, etc.
- See Ruschemeier and Bareis, Gsenger/Sekwenz (eds), Digital Decade: How the EU shapes digitalisation research, Nomos 2025.
- See, e.g., https://en.idi.org.il/articles/6972.
- See, e.g., https://blogs.timesofisrael.com/ive-been-fighting-for-womens-rights-in-israel-for-20-years/ and https://forward.com/life/133065/in-israel-attempts-to-remove-women-from-the-public/.